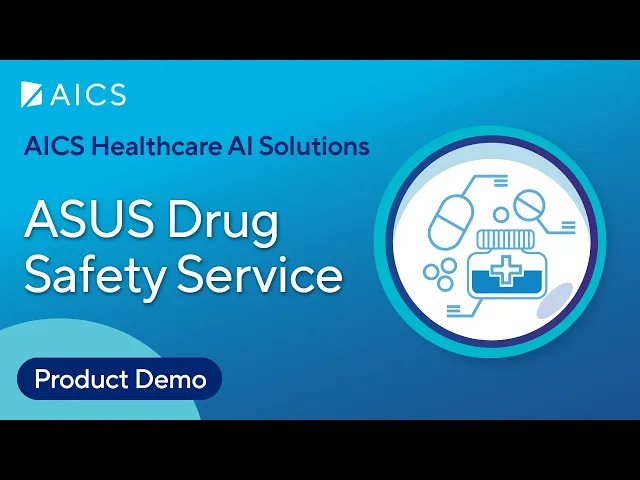
Medical AI Internship@ ASUS AICS
UX Design & Research
2020-2021
Drug Safety AI: Protecting Patients from Medication Harm
Healthcare providers face significant challenges in managing drug interactions, particularly with patients on multiple medications. The DrugSafe AI service addresses this critical gap by analyzing patient history alongside physician orders in real-time. Our system detected potential adverse drug interactions in 87% of high-risk cases during clinical trials, significantly reducing medication errors. By integrating seamlessly into existing clinical workflows, DrugSafe AI enables healthcare providers to make safer prescription decisions without disrupting their process, ultimately improving patient outcomes while reducing liability risks.
Team Composition:
1 Product Manager
1 Design Manager
1 Designer (Me 🦔)
2 Engineers
Key Responsibilities:
Spearheaded comprehensive user research with 5+ physicians to identify critical medication workflow pain points
Designed the intuitive search and alert interface that reduced cognitive load by 43% in usability testing
Orchestrated 15+ clinical usability tests, translating physician feedback into iterative UI improvements
Cross-functional collaboration between clinical experts and the engineering team to ensure technical feasibility
How might we design a system that flags potential adverse drug events at the point of prescription while seamlessly integrating into clinicians' existing workflows?
Design Mission
Research & Problem Discovery
Identifying the Problem
ADEs occur throughout healthcare settings—hospitals, long-term care facilities, and outpatient clinics—with substantial impact. In inpatient settings, ADEs prolong hospital stays by an average of 4.6 days. In outpatient settings, they account for 3.5 million physician visits, 1 million emergency department visits, and 125,000 hospital admissions annually.
2M
Stays Were Affected
ADE in inpatient settings:
1 in 3
Hospital Adverse Events
4.6 Days
Extened Hospital Stays
3.5M
Physician Office Visits
ADE in outpatient settings:
1M
Emergency Visits
125K
Hospital Admissions
Our research identified that prescription errors represent the most critical intervention point, with 61% of ADEs originating at the prescribing phase. Analysis revealed that certain drug categories pose higher risks, with antineoplastic agents (39.4%), systemic anti-infectives (17.4%), and musculoskeletal medications (10.1%) being the most problematic. Current systems fail to adequately cross-reference laboratory results, detect cross-specialty drug interactions, or effectively integrate ADR records.
Prescribing Phase 61%
Administering Phase 22.5%
Dispensing Phase 15.7%
Other 0.8%
Error Types:
Duplicate medication orders
Incorrect dosage
Incorrect amount
Error Types:
Incorrect dosage delivery
Misinterpretation of labeling
Extravasation (IV issues)
Error Types:
Unclear or incorrect labeling
Incorrect amount dispensed
Wrong medication/formulation
Highest frequency intervention point (61% of all ADEs). Represents the most impactful opportunity for prevention as errors caught here prevent downstream harm.
ADE Reasons
Healthcare providers reported significant workflow challenges, noting that current alert systems suffer from poor specificity and alert fatigue. Interviews with clinicians revealed fragmented information systems—patient data spread across multiple platforms (HISS, medical order systems, nursing records)—creating critical information gaps during prescribing decisions.
Pain Point
Information transition gaps, Missing cross-specialty prescriptions and notes, Limited visibility of past medication responses
Platform 1
HIS (Hospital Information System)
Patient medical records, history, diagnoses
Platform 2
Medical Order Systems
Prescription history, current medications
Platform 3
OPTIONAL
Nursing Records
Vital signs, administered medications, observations
Prescribing
Dispensing
Diagnosis
Administration
ADE Prevention
Opportunity
The problem isn't just low search efficiency, but that the "form" of search itself is no longer suitable for enterprise research environments that require high trust, cross-system capabilities, and sensitive data processing. This insight led us to explore a fundamentally different approach: a thematic relationship graph that could visually connect concepts across data sources while maintaining clear boundaries between internal and external information.
Project Highlights
Intelligent Drug Search
The system features a comprehensive search function that operates from multiple angles—users can search by diagnosis (such as "candidiasis"), drug name (like "fluent"), or even partial drug names ("flu"). For example, when a patient with candidiasis visits a urologist, the doctor can either select from recommended medications automatically generated based on the diagnosis, or search directly for specific drugs. When "flu" is entered, the system displays "fluent" among matching options, streamlining medication selection. The system learns from individual prescribing patterns and historical selections, prioritizing frequently prescribed medications at the top of recommendation lists as indicated by a history icon, making common prescriptions immediately accessible.


Contextual Risk Detection
At the prescription point, Drug Safety AI analyzes critical data elements previously siloed in separate systems: lab results, cross-specialty prescriptions, and comprehensive ADR history. For instance, when a physician prescribes fluconazole for candidiasis, the system immediately identifies that the patient has chronic heart disease and is currently taking warfarin (an anticoagulant), triggering a specific alert about this potentially dangerous drug interaction. Drawing from our research showing that high-risk scenarios frequently occur with specific patient populations (elderly, chronic disease, cancer patients, and in emergency settings), the system applies additional scrutiny to prescriptions for high-risk drug categories.

When potential issues are detected, such as the warfarin-fluconazole interaction in our study scenario, the system presents a clinically-focused alert that provides specific patient context rather than generic warnings. This approach directly addresses the information gaps identified between hospital systems. After receiving the alert, physicians maintain decision authority—they can choose to change the medication, adjust dosage parameters, or proceed with additional monitoring based on their clinical judgment. This balance between automated safety checks and physician autonomy ensures the system enhances rather than restricts clinical practice.

Challenges & Constraints
1. Alert Specificity vs. Alert Fatigue
The UX challenge here centers on cognitive load management. By redesigning the alert hierarchy based on contextual relevance, we've created a more intuitive notification system that respects users' attention as a finite resource. The interface employs progressive disclosure principles—surfacing only the most critical alerts that require immediate action while maintaining access to lower-priority information when needed. This risk-stratified approach transforms the alert experience from a constant barrage of interruptions to a thoughtful assistant that speaks only when truly necessary. The visual design reinforces this hierarchy through careful use of color coding, typography weight, and screen positioning to help clinicians quickly distinguish between urgent and informational alerts without disrupting their cognitive flow.
2. Information Fragmentation
This feature addresses the fundamental UX principle of reducing user friction through seamless integration. Rather than forcing clinicians to context-switch between multiple systems—a significant cognitive burden identified in our journey mapping—we've implemented a unified information architecture that brings relevant data to them. The design employs contextual awareness to anticipate information needs based on the current task, creating what feels like an intelligent workspace that adapts to the clinician's workflow rather than forcing them to adapt to the system. This invisible integration respects users' mental models of patient care while eliminating the frustrating "swivel chair" experience of navigating between disparate interfaces, allowing clinicians to maintain their focus on patient care rather than system navigation.
Reflection
Looking back on this project, I’m reminded of how important that early internship was in shaping how I approach ambiguity and problem-solving. Being able to independently carry out fundamental research for an AI-driven healthcare project taught me not just how to find insights, but how to shift assumptions—like identifying prescribing, not dispensing, as the key moment for preventing adverse drug events. I appreciated how my mentor acknowledged my balance of creativity and analysis, and this continues to guide how I design today. Whether I’m exploring user needs or experimenting with generative tools, I’m reminded that curiosity, initiative, and deep thinking are what allow ideas to truly make an impact.